AI Research is not Magic, it has to be Reproducible and Responsible: Challenges that PhD Students in AI face during their Research
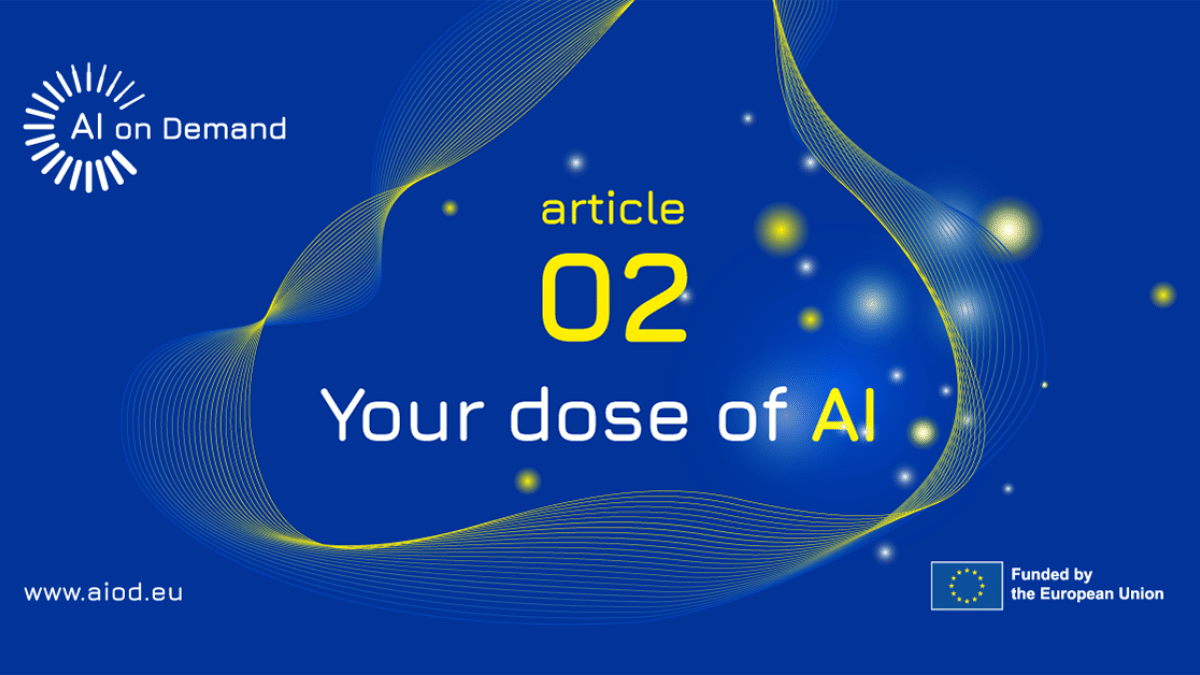
In our study, 28 PhD students from 13 countries across Europe reveal many challenges, including issues with data and code quality, meaning that AI research is facing challenges in reproducing results. If AI findings aren’t reproducible, the entire foundation of technologies built on them becomes shaky—and that has real-world impacts on other fields. By tackling these issues head-on, we can make a shift toward more responsible, transparent and reliable AI.
Five key challenges facing AI research according to the results of the interviews with doctoral students in AI:
- Data Quality is a Major Roadblock: AI researchers reported frequent issues with dataset quality and accessibility, which can undermine research outcomes. Missing expert data annotations, limited access because of privacy hurdles are common and time-consuming challenges that researchers face before even beginning their experiments.
- Reproducibility is in Crisis: A lack of reproducibility means that it’s often impossible to verify the results of AI studies reliably. Researchers found mismatches between published code and experiment results, incomplete documentation, and even errors that make it hard to replicate findings. Thus, the problem of availability of AI assets shifted to the issues of their quality.
- Code and Model Bugs Slow Progress: In addition to dataset issues, many researchers reported encountering bugs in publicly shared code and model inconsistencies that weren’t flagged in the original papers. This not only wastes valuable time but also decreases confidence in shared AI resources.
- Limited Interdisciplinary Collaboration: AI research may benefit greatly from interdisciplinary input, but many early-career researchers found it difficult to connect with experts in other fields. This isolation can limit the robustness and trustworthiness of AI applications applied in other domains.
- Lack of User Research and Human Involvement: Early-stage AI researchers often overlook involving real users or stakeholders in the development process, although the impact of AI on humans is critical. This gap means that user experience, ethical considerations, and practical relevance may be under-addressed, risking AI systems that may not meet real-world needs or expectations. Integrating user research and stakeholder input is essential for creating AI solutions that are both effective and trustworthy.
Solutions for Building a Responsible and Reproducible Future for AI
To address these challenges, we suggest both technical and social solutions to make AI research more robust and trustworthy.
- AI conferences and journals should widely adopt reproducibility checklists and badges, giving clear incentives for sharing reproducible code and data.
- Research institutions could also provide dedicated resources—like standardized coding guidelines or full-time staff for code maintenance—to make high-quality, consistent documentation a norm. Interdisciplinary collaboration, involving ethicists, legal experts, information specialists, user-experience and other specialists is another key recommendation that can more directly address the social and ethical dimensions of technology.
- On the international level, establishing a “cloud federation” of shared resources, where researchers can access standardized tools, data, and models through centralized, reliable infrastructures, will help ensure that AI resources are accessible, reproducible, and relevant for a wider community. And this is where AIoD can help not only doctoral students, but AI researchers in general.
These solutions are setting the foundation for an AI research ecosystem that’s transparent, responsible, and aligned with society’s needs. While technology can help address certain challenges, the bigger hurdle lies in implementing changes at the social level. We need systematic procedural updates, but unfortunately, change management today is often reactive, inconsistent, and unstructured – leading to a high rate of failure. Nevertheless, it is not impossible and the first step is knowing what needs to be changed.
Author: Andrea Hrčková (KInIT)